TensorFlow Serving¶
當我們將模型訓練完畢後,往往需要將模型在開發環境中部署。最常見的方式是在伺服器上提供一個 API,即客戶端向伺服器的某個 API 發送特定格式的請求,伺服器收到請求資料後透過模型進行計算,並返回結果。如果只是做一個 Demo,不考慮並發性和性能問題,其實配合 Flask 等 Python 下的 Web 框架就能非常輕鬆地實現伺服器 API。不過,如果是在真的實際開發環境中部署,這樣的方式就顯得力不從心了。這時,TensorFlow 為我們提供了 TensorFlow Serving 這一組件,能夠幫助我們在實際開發環境中靈活且高性能地部署機器學習模型。
TensorFlow Serving 安裝¶
TensorFlow Serving 可以使用 apt-get 或 Docker安裝。在開發環境中,推薦 使用Docker部署TensorFlow Serving 。不過此處出於教學目的,介紹環境依賴較少的 apt-get安裝 。
警告
軟體的安裝方法往往具有時效性,本節的更新日期為2019年8月。若遇到問題,建議參考 TensorFlow網站上的最新安裝說明 進行操作。
首先設置安裝來源:
# 添加Google的TensorFlow Serving安裝來源
echo "deb [arch=amd64] http://storage.googleapis.com/tensorflow-serving-apt stable tensorflow-model-server tensorflow-model-server-universal" | sudo tee /etc/apt/sources.list.d/tensorflow-serving.list
# 添加gpg key
curl https://storage.googleapis.com/tensorflow-serving-apt/tensorflow-serving.release.pub.gpg | sudo apt-key add -
更新安裝來源後,即可使用 apt-get 安裝 TensorFlow Serving
sudo apt-get update
sudo apt-get install tensorflow-model-server
提示
在執行 curl 和 apt-get 命令時,可能需要設置代理伺服器。
curl 設置代理伺服器的方式為 -x
選項或設置 http_proxy
環境變量,即
export http_proxy=http://代理伺服器IP:埠號
或
curl -x http://代理伺服器IP:埠號 URL
apt-get設置代理的方式為 -o
選項,即
sudo apt-get -o Acquire::http::proxy="http://代理伺服器IP:埠號" ...
Windows 10 下,可以在 Linux子系統(WSL) 使用相同的方式安裝 TensorFlow Serving。
TensorFlow Serving 模型部署¶
TensorFlow Serving 可以直接讀取 SavedModel 格式的模型進行部署(匯出模型到 SavedModel 文件的方法見 前文 )。使用以下指令即可:
tensorflow_model_server \
--rest_api_port=埠號(如8501) \
--model_name=模型名 \
--model_base_path="SavedModel格式模型的資料夾絕對地址(不含版本號碼)"
注解
TensorFlow Serving 支援即時更新模型,其典型的模型資料夾結構如下:
/saved_model_files
/1 # 版本號碼為1的模型文件
/assets
/variables
saved_model.pb
...
/N # 版本號碼為N的模型文件
/assets
/variables
saved_model.pb
上面 1~N 的子資料夾代表不同版本號的模型。當指定 --model_base_path
時,只需要指定根目錄的 絕對路徑 (不是相對路徑)即可。例如,如果上述資料夾結構存放在 home/snowkylin
資料夾內,則 --model_base_path
應該設置為 home/snowkylin/saved_model_files
(不附帶模型版本號碼)。TensorFlow Serving 會自動選擇版本號碼最大的模型進行載入。
Keras Sequential 模式模型的部署¶
由於 Sequential 模式的輸入和輸出都很固定,因此這種類型的模型很容易部署,不需要其他額外操作。例如,要將 前文使用 SavedModel 匯出的 MNIST 手寫體識別模型 (使用Keras Sequential模式建立)以 MLP
的模型名在埠號 8501
進行部署,可以直接使用以下指令:
tensorflow_model_server \
--rest_api_port=8501 \
--model_name=MLP \
--model_base_path="/home/.../.../saved" # 資料夾絕對路徑根據自身情況填寫,無需加入版本號碼
然後就可以按照 後文的介紹 ,使用 gRPC 或者 RESTful API 在客戶端呼叫模型了。
自定義 Keras 模型的部署¶
使用繼承 tf.keras.Model
類建立的自定義 Keras 模型的自由度相對更高。因此使用 TensorFlow Serving 部署模型時,對導出的 SavedModel 文件也有更多的要求:
匯出 SavedModel 格式的方法(比如
call
)不僅需要使用@tf.function
修飾,還要在修飾時指定input_signature
參數,以說明輸入的形狀。該參數傳入一個由tf.TensorSpec
組成的列表,指定每個輸入張量的形狀和類型。例如,對於 MNIST 手寫體數字辨識,我們的輸入是一個[None, 28, 28, 1]
的四維張量(None
表示第一維即 Batch Size 的大小不固定),此時我們可以將模型的call
方法做以下修飾:
class MLP(tf.keras.Model):
...
@tf.function(input_signature=[tf.TensorSpec([None, 28, 28, 1], tf.float32)])
def call(self, inputs):
...
將模型使用
tf.saved_model.save
匯出時,需要通過signature
參數提供待匯出的函數的簽名(Signature)。簡單說來,由於自定義的模型類別裡可能有多個方法都需要匯出,因此,需要告訴 TensorFlow Serving 每個方法在被客戶端呼叫時分別叫做什麼名字。例如,如果我們希望客戶端在呼叫模型時使用call
這一簽名來呼叫model.call
方法時,我們可以在匯出時傳入signature
參數,以dict
的形式告知匯出的方法對應的名稱,程式碼如下:
model = MLP()
...
tf.saved_model.save(model, "saved_with_signature/1", signatures={"call": model.call})
以上兩步均完成後,即可使用以下指令部署:
tensorflow_model_server \
--rest_api_port=8501 \
--model_name=MLP \
--model_base_path="/home/.../.../saved_with_signature" # 修改為自己模型的絕對地址
在客戶端呼叫以 TensorFlow Serving 部署的模型¶
TensorFlow Serving 支援以 gRPC和RESTful API 調用以 TensorFlow Serving 部署的模型。本手冊主要介紹較為通用的 RESTful API 方法。
RESTful API 以標準的 HTTP POST 方法進行通信,請求和回覆均為 JSON 對象。為了呼叫伺服器端的模型,我們在客戶端向伺服器發送以下格式的請求:
伺服器 URI: http://伺服器地址:埠號/v1/models/模型名:predict
請求內容:
{
"signature_name": "需要呼叫的函數簽名(Sequential模式不需要)",
"instances": 輸入資料
}
回覆為:
{
"predictions": 返回值
}
Python 客戶端範例¶
以下範例使用 Python的Requests庫 (你可能需要使用 pip install requests
安裝該函式庫)向本機的TensorFlow Serving 伺服器發送 MNIST 測試集的前 10 幅圖像並返回預測結果,同時與測試集的真實標籤進行比較。
import json
import numpy as np
import requests
from zh.model.utils import MNISTLoader
data_loader = MNISTLoader()
data = json.dumps({
"instances": data_loader.test_data[0:3].tolist()
})
headers = {"content-type": "application/json"}
json_response = requests.post(
'http://localhost:8501/v1/models/MLP:predict',
data=data, headers=headers)
predictions = np.array(json.loads(json_response.text)['predictions'])
print(np.argmax(predictions, axis=-1))
print(data_loader.test_label[0:10])
輸出:
[7 2 1 0 4 1 4 9 6 9]
[7 2 1 0 4 1 4 9 5 9]
可以發現,預測結果與真實標籤值非常接近。
對於自定義的 Keras 模型,在發送的資料中加入 signature_name
鍵值即可,將上面程式碼 data
建立過程改為
data = json.dumps({
"signature_name": "call",
"instances": data_loader.test_data[0:10].tolist()
})
Node.js客戶端範例(Ziyang)¶
以下範例使用 Node.js 將下圖轉換為 28*28 的灰階圖,發送給本機的 TensorFlow Serving 伺服器,並輸出返回的預測結果和機率。(其中使用了 圖像處理庫jimp 和 HTTP庫superagent ,可使用 npm install jimp
和 npm install superagent
安裝)
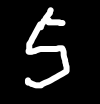
test_pic_tag_5.png
:一個由作者手寫的數字5。(運行下麵的代碼時可下載該圖片並放在與代碼同一目錄下)¶
const Jimp = require('jimp')
const superagent = require('superagent')
const url = 'http://localhost:8501/v1/models/MLP:predict'
const getPixelGrey = (pic, x, y) => {
const pointColor = pic.getPixelColor(x, y)
const { r, g, b } = Jimp.intToRGBA(pointColor)
const gray = +(r * 0.299 + g * 0.587 + b * 0.114).toFixed(0)
return [ gray / 255 ]
}
const getPicGreyArray = async (fileName) => {
const pic = await Jimp.read(fileName)
const resizedPic = pic.resize(28, 28)
const greyArray = []
for ( let i = 0; i< 28; i ++ ) {
let line = []
for (let j = 0; j < 28; j ++) {
line.push(getPixelGrey(resizedPic, j, i))
}
console.log(line.map(_ => _ > 0.3 ? ' ' : '1').join(' '))
greyArray.push(line)
}
return greyArray
}
const evaluatePic = async (fileName) => {
const arr = await getPicGreyArray(fileName)
const result = await superagent.post(url)
.send({
instances: [arr]
})
result.body.predictions.map(res => {
const sortedRes = res.map((_, i) => [_, i])
.sort((a, b) => b[0] - a[0])
console.log(`我們猜這個數字是${sortedRes[0][1]},機率是${sortedRes[0][0]}`)
})
}
evaluatePic('test_pic_tag_5.png')
執行結果為:
1 1 1 1 1 1 1 1 1 1 1 1 1 1 1 1 1 1 1 1 1 1 1 1 1 1 1 1
1 1 1 1 1 1 1 1 1 1 1 1 1 1 1 1 1 1 1 1 1 1 1 1 1 1 1 1
1 1 1 1 1 1 1 1 1 1 1 1 1 1 1 1 1 1 1 1 1 1 1 1 1 1
1 1 1 1 1 1 1 1 1 1 1 1 1 1 1 1 1 1 1 1 1 1 1 1 1 1
1 1 1 1 1 1 1 1 1 1 1 1 1 1 1 1 1 1 1 1 1 1 1 1 1 1
1 1 1 1 1 1 1 1 1 1 1 1 1 1 1 1 1 1 1 1 1
1 1 1 1 1 1 1 1 1 1 1 1 1 1 1 1 1 1 1 1
1 1 1 1 1 1 1 1 1 1 1 1 1 1 1 1 1 1 1 1 1 1 1 1 1 1
1 1 1 1 1 1 1 1 1 1 1 1 1 1 1 1 1 1 1 1 1 1 1 1 1 1
1 1 1 1 1 1 1 1 1 1 1 1 1 1 1 1 1 1 1 1 1 1 1 1 1
1 1 1 1 1 1 1 1 1 1 1 1 1 1 1 1 1 1 1 1 1 1 1 1 1 1
1 1 1 1 1 1 1 1 1 1 1 1 1 1 1 1 1 1 1 1 1 1 1 1 1
1 1 1 1 1 1 1 1 1 1 1 1 1 1 1 1 1 1
1 1 1 1 1 1 1 1 1 1 1 1 1 1 1 1
1 1 1 1 1 1 1 1 1 1 1 1 1 1 1 1 1 1 1 1 1 1
1 1 1 1 1 1 1 1 1 1 1 1 1 1 1 1 1 1 1 1 1 1 1 1 1 1
1 1 1 1 1 1 1 1 1 1 1 1 1 1 1 1 1 1 1 1 1 1 1 1 1 1
1 1 1 1 1 1 1 1 1 1 1 1 1 1 1 1 1 1 1 1 1 1 1 1 1
1 1 1 1 1 1 1 1 1 1 1 1 1 1 1 1 1 1 1 1 1 1 1 1 1 1
1 1 1 1 1 1 1 1 1 1 1 1 1 1 1 1 1 1 1 1 1 1 1 1
1 1 1 1 1 1 1 1 1 1 1 1 1 1 1 1 1 1 1 1 1 1 1 1
1 1 1 1 1 1 1 1 1 1 1 1 1 1 1 1 1 1 1 1 1 1
1 1 1 1 1 1 1 1 1 1 1 1 1 1 1 1 1 1 1 1
1 1 1 1 1 1 1 1 1 1 1 1 1 1 1 1 1 1 1 1 1 1 1 1
1 1 1 1 1 1 1 1 1 1 1 1 1 1 1 1 1 1 1 1 1 1 1 1 1 1 1 1
1 1 1 1 1 1 1 1 1 1 1 1 1 1 1 1 1 1 1 1 1 1 1 1 1 1 1 1
1 1 1 1 1 1 1 1 1 1 1 1 1 1 1 1 1 1 1 1 1 1 1 1 1 1 1 1
1 1 1 1 1 1 1 1 1 1 1 1 1 1 1 1 1 1 1 1 1 1 1 1 1 1 1 1
我們猜這個數字是5,機率是0.846008837
可以發現、輸出結果符合預期。